Understanding how pricing impacts profitability is crucial for businesses.
Pricing analytics uses data to provide insights into customer behavior, market trends, and competitor strategies — ultimately helping businesses set prices that maximize revenue and meet customer expectations.
This guide will explore what pricing analytics is, its benefits, and how businesses can use it to gain a competitive edge, boost customer satisfaction, and drive profitability.
What is pricing analytics?
Pricing analytics is the process of using data and analytics to understand how pricing impacts a business and take a strategic, data-driven approach to decision-making.
Companies can use data to inform their pricing strategy by analyzing competitor pricing, market trends, and customer behavior.
“Pricing analytics is using data to set prices in a way that maximizes revenue and improves profit,” Tom Haylock, CEO of Sharecat, says. “It’s not just a matter of setting prices high or low; it’s about finding that sweet spot where demand meets profitability.”
The importance of pricing analytics
Pricing analytics is a strategic approach using data to set optimal price points, maximize revenue, and meet customer needs, and develop competitive pricing.
Businesses can create tailored pricing structures by understanding customer preferences, sensitivity to pricing, and willingness to pay.
Benefits of pricing analytics
Using data-driven insights helps businesses create an effective pricing strategy and make informed decisions about pricing changes.
Additional benefits of pricing analytics include:
Increased profitability
Pricing analytics helps businesses identify optimal price points to attract customers and maximize revenue without overpricing or underpricing.
“Effective pricing analytics is like creating precision-engineered tags – it requires careful attention to detail and continuous refinement based on data,” David Primrose, President at Metal Marker Manufacturing, says. “By understanding your customers’ perceived value and willingness to pay, you can develop pricing strategies that boost profitability.”
Enhanced audience segmentation and customized pricing
Understanding how different customer segments respond to specific pricing strategies can help you refine your product pricing to cater to your customers’ needs.
“Price analytics helps you segment your audience better; through data analysis, you get insights that guide your market segmentation.” David Kemmerer, Co-Founder and CEO CoinLedger, says. “When we did our price analytics, we discovered small businesses were most sensitive to pricing, and larger companies were less sensitive to price but cared more about features our software offers and supports.”
Using pricing data empowers businesses to design pricing plans that cater to different demographics and customer segments, balancing affordability with premium options for various needs.
Companies can offer dynamic pricing strategies that adapt to customer needs and market conditions, such as tiered pricing, discounts, or bundling.
“Not all customers have high purchasing power — some are willing to pay for premium features, while others value affordability,” Stefan Chekanov, Co-Founder and CEO of Brosix, says. “Pricing analytics shows which segments are the most profitable and how different groups react to price changes. Once you know this information, you can design specific pricing plans that fit each type of customer.”
Creating customized pricing for different customer segments can be challenging. With PandaDoc CPQ, sales teams can use dynamic pricing models and rule engines to create fast, accurate quotes.
PandaDoc also integrates with CRM tools like HubSpot and Salesforce to make it easier for your team to streamline customer data and create dynamic pricing.
Reduced churn and increased retention
Pricing analytics can help companies identify reasons for customer churn, like high prices or perceived lack of value. Armed with this data, companies can make adjustments to retain customers.
“Pricing analytics isn’t a guessing game; it’s strategically using data to see how customers respond to each price point,” Austin Rulfs, Director of Zanda Wealth, says. “You can look at factors like conversion rates, churn, and how long customers stick around to find that sweet spot where they feel they’re getting real value, and you’re seeing strong returns. For example, if you’re noticing a lot of churn at the lower tiers, it could mean customers aren’t finding enough value there, and it might be time to rethink what you’re offering or try more customized tiers.”
Competitive advantage
Using data sets allows companies to identify pricing opportunities and make adjustments to keep companies aligned with market trends and competitive with other offerings.
“It’s essential for staying competitive,” Stefan says.” With pricing insights, businesses can see how their prices stack up against others in the market.”
Why pricing analytics is important for SaaS companies
Pricing analytics is particularly important for SaaS companies because the subscription-based revenue model requires ongoing customer retention and long-term relationships to drive profitability.
Unlike industries where revenue depends on one-time purchases or product lines, SaaS revenue is generated over time through monthly or annual subscriptions.
Reducing churn is essential to minimizing the impact on recurring revenue.
Key reasons why pricing analytics is critical for Saas:
Diagnose and prevent churn
Pricing analytics helps to identify and counteract the reasons customers leave, including high prices, lack of perceived value, or usability issues.
Lifetime value and customer acquisition cost (CAC)
Pricing analytics helps refine pricing to maximize LTV while keeping CAC manageable, which is vital when growth hinges on retaining customers over long periods.
Scalability and flexibility
SaaS products often evolve quickly, adding new features or modifying existing ones.
Pricing analytics helps companies adjust prices and tiers as the product changes, ensuring that each feature set aligns with customer expectations and market demand without underpricing or overpricing the offering.
How pricing analytics improves profitability
Pricing analytics improves profitability by enabling businesses to set prices strategically using data-driven insights into customer behavior, competitor data, product value, and market demand.
Armed with this data, businesses can determine the best price points for their products or services based on various conditions to maximize their profits.
Organizations can also use historical sales data, customer behavior, and market trends to forecast changes and adjust their prices.
Analyzing this data helps businesses capitalize on high demand or other trends to find the optimal pricing strategy.
Because pricing analytics enables better customer segmentation, organizations can offer different pricing for different customers, which will benefit both the customers and the business.
“Pricing analytics involves using data to optimize pricing strategies, helping businesses understand how price changes impact revenue and demand,” Deepak Shukla, Founder of Pearl Lemon Leads, says. “It improves profitability by identifying the best price points, optimizing discounts, and forecasting market demand.”
Types of pricing analytics
In this section, we’ll explore the primary types of pricing analytics — descriptive, predictive, and prescriptive — that empower businesses to make data-informed pricing decisions.
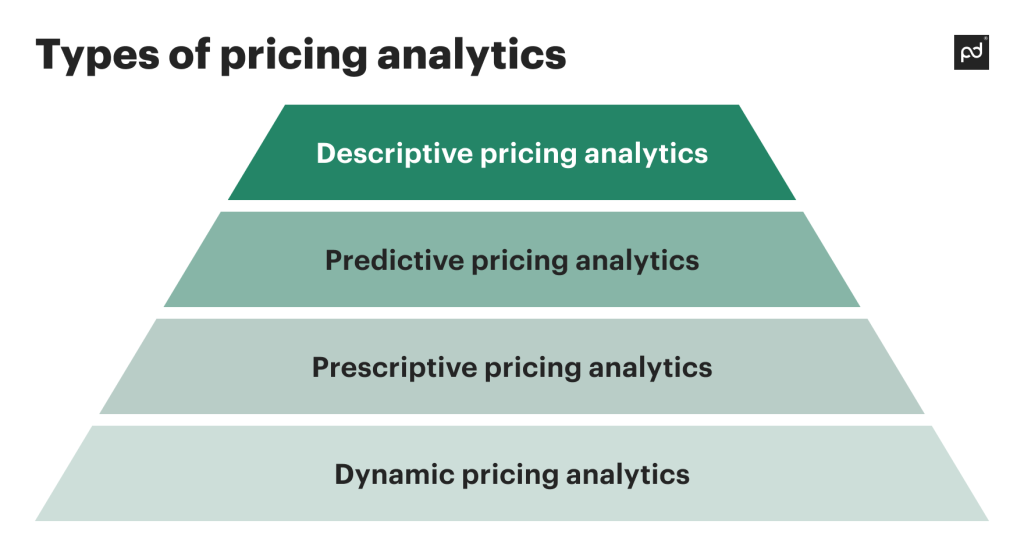
Descriptive pricing analytics
Descriptive pricing analytics uses historical data to understand customer behavior in response to pricing changes.
Descriptive pricing analytics involves identifying trends from past sales, pricing tiers, and customer segments to analyze what worked and why.
To use this strategy, businesses may analyze:
- Month-over-month or year-over-year price growth
- Year-over-year price changes
- Average revenue per customer
Descriptive pricing analytics can also be used to identify trends in customer behavior. Streaming platform Netflix uses descriptive analytics in its trending now feature.
More than 80% of the shows people watch on the platform are discovered through the recommendation system.
This method offers a baseline for predictive and prescriptive analyses to refine and optimize pricing decisions.
Prescriptive pricing analytics
While descriptive pricing analytics identifies trends in historical data, prescriptive pricing analytics is more forward-looking and actionable.
Prescriptive pricing analytics uses algorithms and machine learning to establish pricing plans and help businesses understand how pricing changes can affect future performance.
It analyzes data, recommends optimal pricing strategies, and adjusts to real-time changes in demand, competition, and customer behavior.
Retail businesses use prescriptive analytics to predict product demand and adjust prices accordingly. They use historical sales data and past seasonal trends to determine their inventory and prices.
Predictive pricing analytics
Predictive pricing analytics combines historical data, algorithms, and machine learning to predict outcomes as pricing changes.
This model aims to predict trends like customer demand, price sensitivity, and revenue potential at different price points.
Retail businesses use predictive pricing analytics to forecast optimal product prices based on anticipated demand, competitor pricing, and seasonal trends.
By analyzing historical sales data and real-time factors, they can adjust prices dynamically to maximize profits, attract price-sensitive customers, and manage inventory effectively.
Type | How it works |
---|---|
Descriptive | Uses historical data to understand customer behavior in response to pricing changes – Identifies trends from past sales, pricing tiers, and customer segments to analyze what worked and why |
Prescriptive | Uses algorithms and machine learning to establish pricing plans – Helps businesses understand how pricing changes can affect future performance – Analyzes data, recommends optimal pricing strategies, and adjusts to real-time changes in demand, competition, and customer behavior |
Predictive | Combines historical data, algorithms, and machine learning to predict outcomes as pricing changes – Predicts trends like customer demand, price sensitivity, and revenue potential at different price points |
Pricing metrics to track
So what are the most important metrics to track to make data-driven decisions and find the right price for your products, service, and customers? We’ll go into what to measure below.
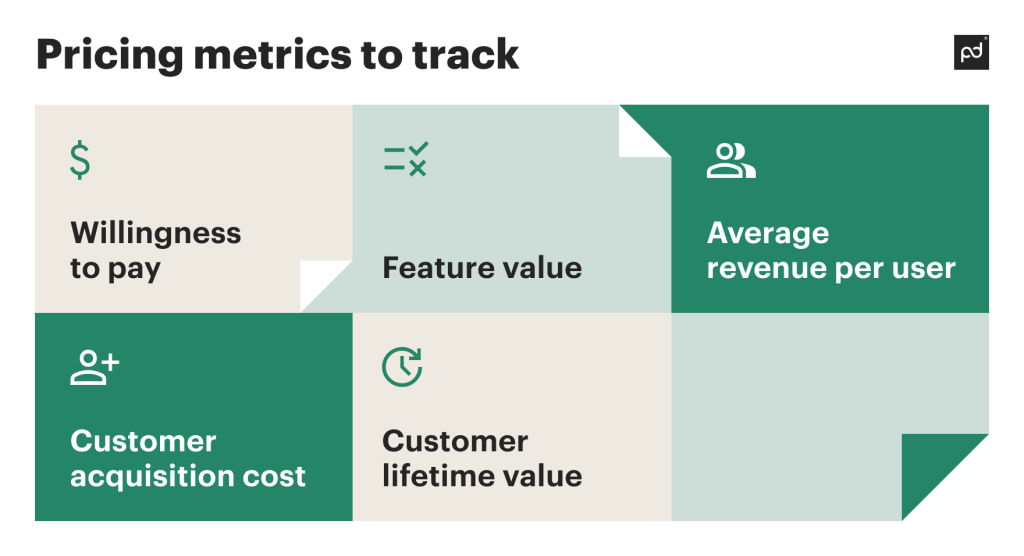
Willingness to pay (WTP)
Willingness to pay is the highest price a customer is willing to pay for a product or service.
WTP generally represents a customer’s perceived value of the product and is a price range vs. a single dollar amount.
Common ways to measure willingness to pay include customer surveys and market research.
Feature value
Just as willingness to pay measures a customer’s perceived value, feature value takes this a step further to measure how much customers value individual features.
You can collect this information by asking customers directly or looking at related metrics like feature adoption and usage frequency.
Feature value is especially helpful for products with tiered pricing structures.
Average revenue per user (ARPU)
Average revenue per user measures how much revenue a company earns from each user over a specified time period.
To calculate ARPU, divide total revenue by the number of users or subscribers.
ARPU = Total Revenue
______________
Number of Users
Customer acquisition cost (CAC)
Customer acquisition cost measures how much it costs to acquire a new customer.
This includes any costs for marketing and advertising, including the tools your team uses and the salaries for these team members, customer service, including tools and salaries for personnel, and overhead.
Use this formula to calculate CAC:
CAC = Total Cost (including sales, marketing, overhead, etc.) / # of customers acquired in the designated time period
Customer lifetime value (CLV)
Customer lifetime value measures the amount of potential revenue generated from a customer. It’s an important metric for helping businesses understand the value of customer retention.
To calculate CLV:
Average number of purchases/orders a customer makes X the average order value
Ideally, your customer lifetime value should be higher than your customer acquisition cost.
Improve profitability with pricing analytics
Pricing analytics is more than just a pricing strategy — it’s a critical tool for building sustainable profitability.
By using data-driven insights, businesses can create tailored pricing structures, track key performance metrics, and adjust pricing dynamically to align with market demand.
Creating customized pricing for different customers and changing your prices to meet market demands can be complicated.
Learn more about how PandaDoc CPQ can help you create a dynamic pricing strategy and generate fast, accurate quotes.
Contact us to learn more and schedule a free, personalized demo.
Disclaimer
PandaDoc is not a law firm, or a substitute for an attorney or law firm. This page is not intended to and does not provide legal advice. Should you have legal questions on the validity of e-signatures or digital signatures and the enforceability thereof, please consult with an attorney or law firm. Use of PandaDoc services are governed by our Terms of Use and Privacy Policy.